Can semiconductors help with reducing the carbon footprint of bitcoin mining and other high-performance computing (HPC) applications? Can the overall compute power needed be reduced? These two questions were a common theme during several June and July industry conferences. The answer to the first question is a resounding “Yes!”, as chips driving more intelligent systems can be used to reduce the carbon footprint across many industries. The second question or theme is considerably more challenging, and one that the semiconductor and computing industry needs to address as computing becomes more ubiquitous.
HPC demands increasingly more energy to process the data. One solution that I have heard proposed at conferences is that if the energy used is from renewables, then it doesn’t matter if HPC consumes more energy. From my viewpoint, this is short-sighted and just kicks the energy can further down the road.
Bitcoin mining has been the recent poster child of HPC energy consumption. The University of Cambridge study has estimated that the annual power consumption of bitcoin is approximately 130 terawatt-hours, which is more than three times higher than at the beginning of 2019. China just recently ordered a halt to bitcoin mining and the sale of bitcoin mining machines, due to concerns over the amount of energy consumed.
With the energy requirements for bitcoin mining being very high, one company reopened a coal-fired power plant to meet their energy demands. An ingenious approach, but not a green one (Figure 1).
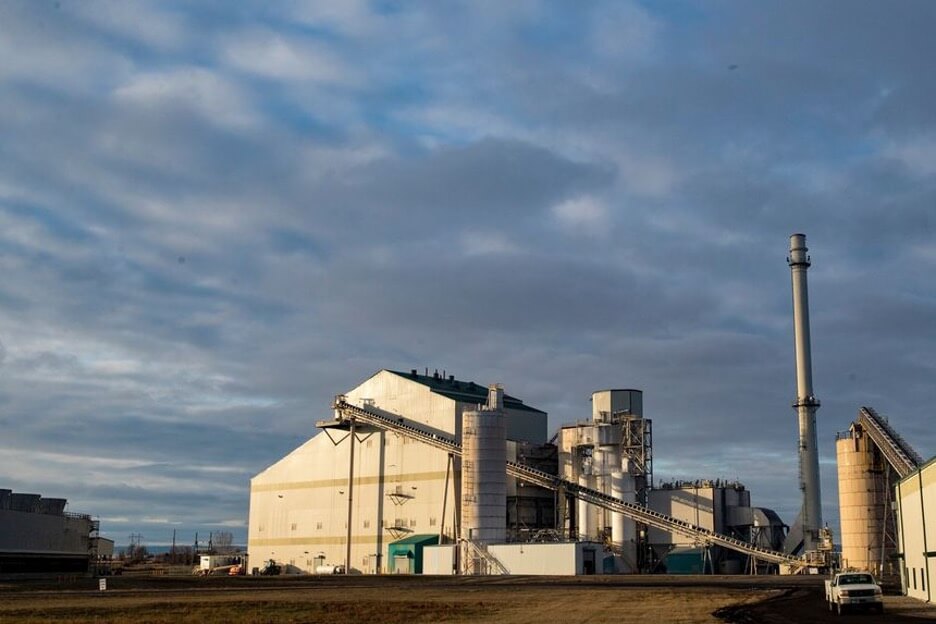
The Impact of AI On Energy Consumption
Bitcoin mining is not the only energy hog in the HPC room. The teach component of artificial intelligence (AI) consumes a considerable amount of energy. Figure 1 by Open AI shows the increasing amount of compute needed to train systems. A couple of other studies, one by the University of Massachusetts, Amherst found that the electricity consumed during the training of a transformer, a type of deep-learning algorithm, can emit more than 626,000 pounds of carbon dioxide. The energy amounts generated vary depending upon the requirements of the teach cycle (Figure 2).
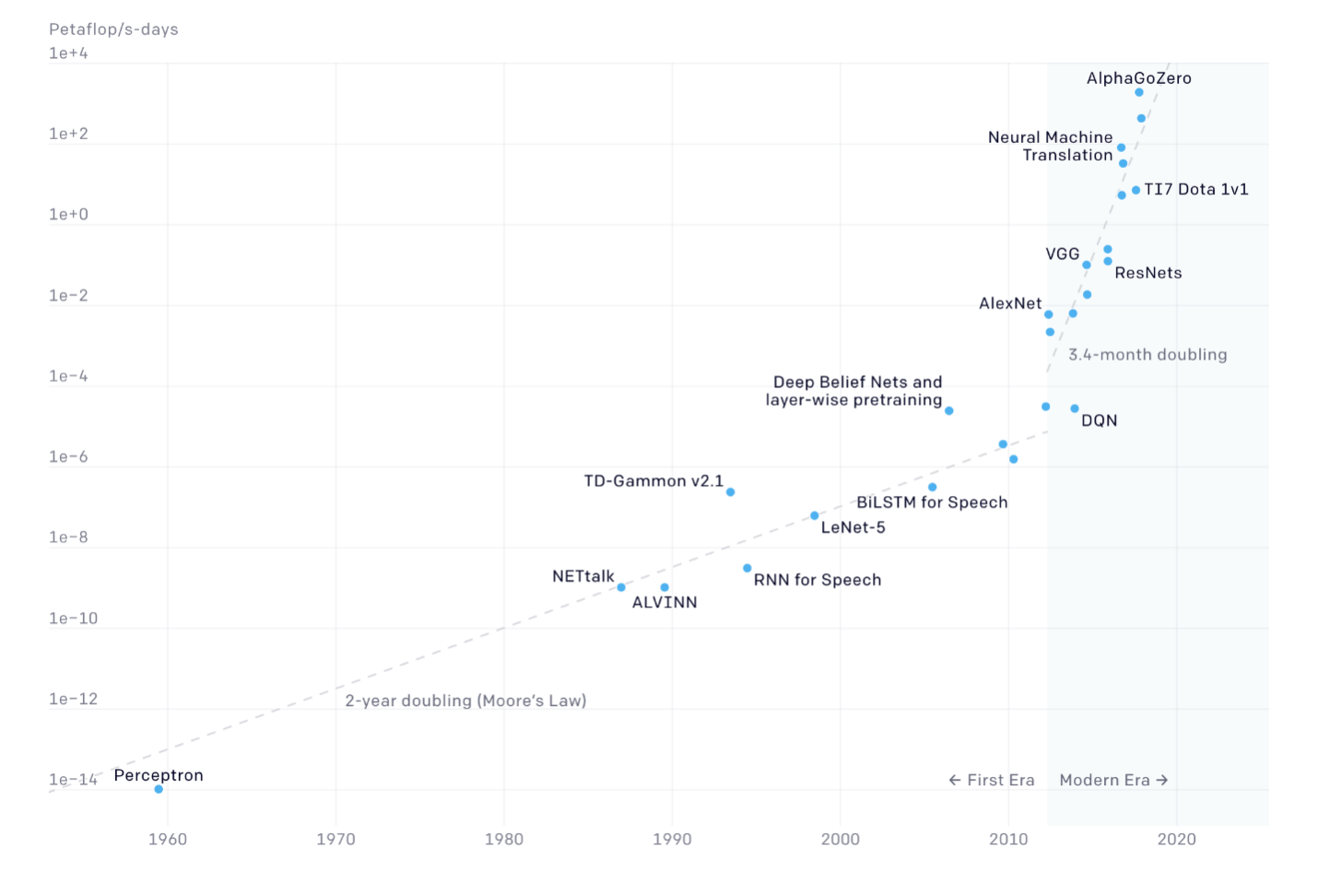
Towards data science performed an interesting breakdown on data centers using CPU and GPU, as currently both are needed for AI as well as data analysis. Their study estimates a data center would use 52.8 MWh/day, in their words – “a scary number”. This study went one step further. As I mentioned earlier, one of the arguments behind increasing HPC energy consumption is that data centers are being run on renewable energy. The towards data science team calculates the number of wind turbines needed to run the 500,000 data centers they estimate exist in the world. The number they arrive at is 2.5 million wind turbines that would be needed to meet the energy demands. When the article was written in 2019, they estimate the US and Germany combined have around 100,000 wind turbines between them, so we have a lot of wind turbines to install to meet the server farm demand.
The above information doesn’t give a favorable outlook for reducing energy consumption or the carbon footprint for HPC. Fortunately, there is a great deal of work ongoing in an attempt to reduce the energy needed to compute.
In the AI space, inference uses considerably less energy than learning. Having said that there are still 500,000 data centers out there that need power. Google has been using AI to find ways to reduce the power consumed by its data centers and reports its considerable success here. Google has reduced the amount of energy needed to cool the data centers by 30-40%.
While this is the first step, it is just the tip of the iceberg, so to speak. The electronics industry also needs to find ways to reduce the amount of energy or power produced in the first place. One of the chief consumers of energy is the movement of data around the system. Logic to memory and back and between other components on the server board. Andrew Fieldman, founder, and CEO of Cerebras comments that using a CPU and GPU for AI training equates to using and 18-wheeler truck to take the kids to soccer practice.
Cerebras along with Google, AWS, Microsoft, and many other companies have been creating chip designs specific for AI learning applications. While the server farm companies are using ASIC designs and heterogenous packaging to combine specific technology together to address AI, Cerebras uses a single chip placed on a 300mm wafer. The Cerebras design reduces the amount of switching that needs to take place between different components, and thus reducing the amount of energy or power that is consumed moving data around the chip. According to Fieldman, the Cerebras design uses a tenth of the power and rack space of a GPU system that would achieve equivalent performance to the Cerebras design.
Building a 300mm ASIC is not without its challenges, which is why others are looking at using 3D packaging as the solution for better performance. There is not a lot of data yet on the AI solutions using 3D packaging technology, but hopefully, the industry is looking at how those solutions can reduce the power consumed by computing, especially as compute and AI move closer to the edge.
If we could figure out a way to reduce compute power by 10x while achieving the same performance, that would go a long way in reducing the carbon footprint of the electronics industry.